Resources > Data Management
3+ Applications of Big Data in Healthcare (Real Examples)
15-Minute Read
April 14, 2025
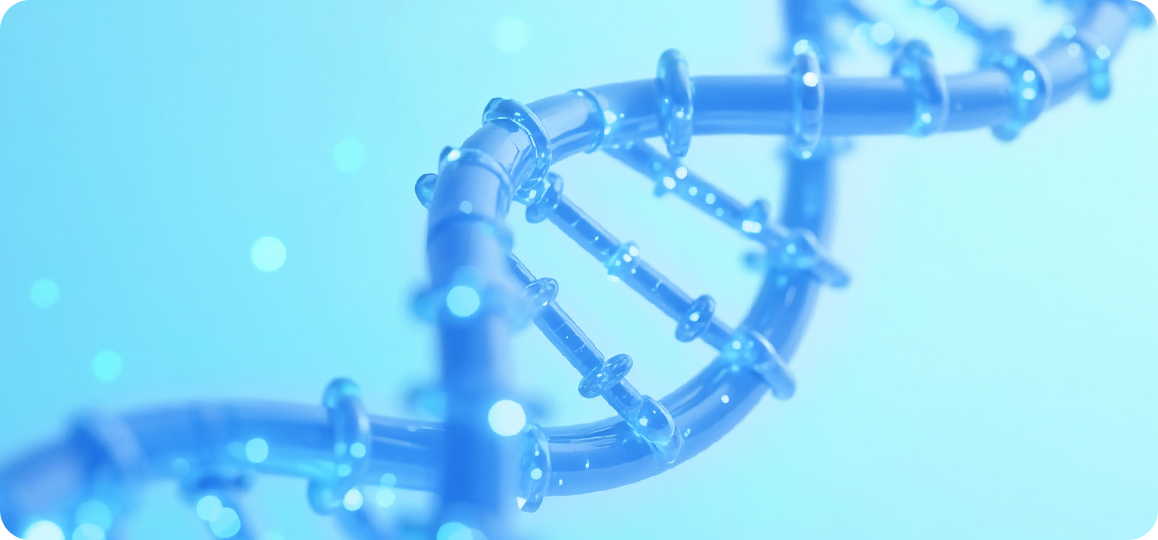
Big data in healthcare encompasses the vast, complex datasets generated from electronic health records (EHRs), wearable devices, genomic sequencing, and medical imaging. Characterized by high volume, velocity, and variety, this data requires advanced analytics to extract meaningful insights.
Research suggests that big data in healthcare can improve patient outcomes, reduce costs, and enhance operational efficiency, making it a cornerstone of modern healthcare transformation. For instance, a 2024 report by McKinsey estimated that big data could save the U.S. healthcare system between $300 billion and $450 billion annually by optimizing operations.
Below, we explore some applications of big data in healthcare and the business impact they offer, from cost savings to improved patient outcomes.
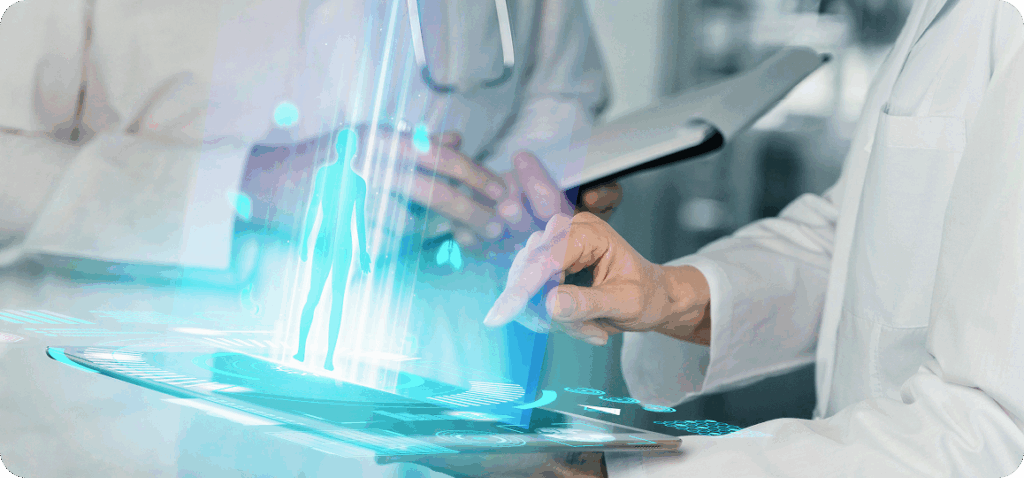
Predictive Analytics for Patient Outcomes
One of the most powerful uses of big data is predictive analytics in healthcare. By analyzing historical and real-time data, health systems can predict future events, such as disease outbreaks, patient deterioration, or readmission risk, and intervene early. This shift from reactive to proactive care can save lives and reduce costs.
For example, New York University researchers recently developed an AI model (NYUTron) that analyzes electronic health records to accurately forecast patient outcomes like hospital readmissions and length of stay. Such tools enable clinicians to identify high-risk patients, allowing preemptive steps to prevent complications.
Another notable example is Google’s DeepMind, which developed an AI system to predict acute kidney injury (AKI) up to 48 hours before it occurs.
In a 2019 study published in Nature, DeepMind collaborated with the U.S. Department of Veterans Affairs, analyzing over 620,000 data points from EHRs to train the model DeepMind’s AI for AKI Prediction.
The AI accurately predicted 90% of severe cases requiring dialysis, demonstrating its potential to prevent avoidable patient harm. This application is particularly valuable for high-risk patients, such as those in intensive care, where timely interventions can be life-saving.
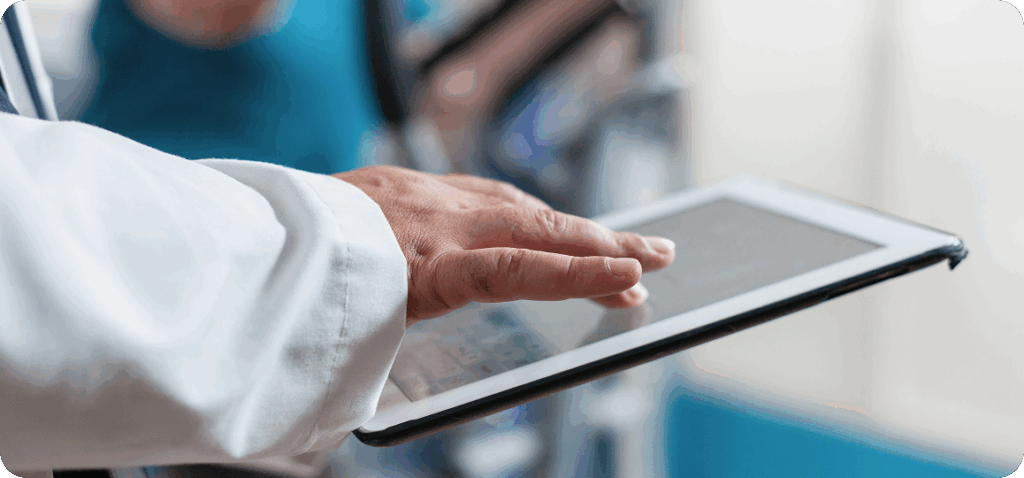
Improving Operational Efficiency in Hospitals
Beyond clinical care, big data is revolutionizing the operational side of healthcare delivery. Large hospitals are complex enterprises, and optimizing staffing, scheduling, supply chains, and resource allocation is a constant challenge.
Big data analytics can uncover patterns in operational workflows and suggest efficiencies that humans might miss.
Hospitals like Massachusetts General Hospital (MGH) have reported significant gains by applying data analytics to operations. MGH used a data-driven approach to analyze patient flow, staffing levels, and equipment use, identifying bottlenecks in real time.
By using advanced techniques (e.g., queuing theory and simulation modeling), they optimized scheduling and resource deployment, resulting in shorter wait times and reduced operational costs.
Another example is Seattle Children’s Hospital, which built a “digital twin” of its hospital operations to run simulations for resource allocation. This predictive modeling allowed administrators to test how changes (like adjusting nurse shifts or operating room schedules) would impact throughput and capacity.
During the COVID-19 pandemic, this capability was critical; Seattle Children’s could forecast how quickly PPE stockpiles might run out and how many ICU beds would be needed under various scenarios.
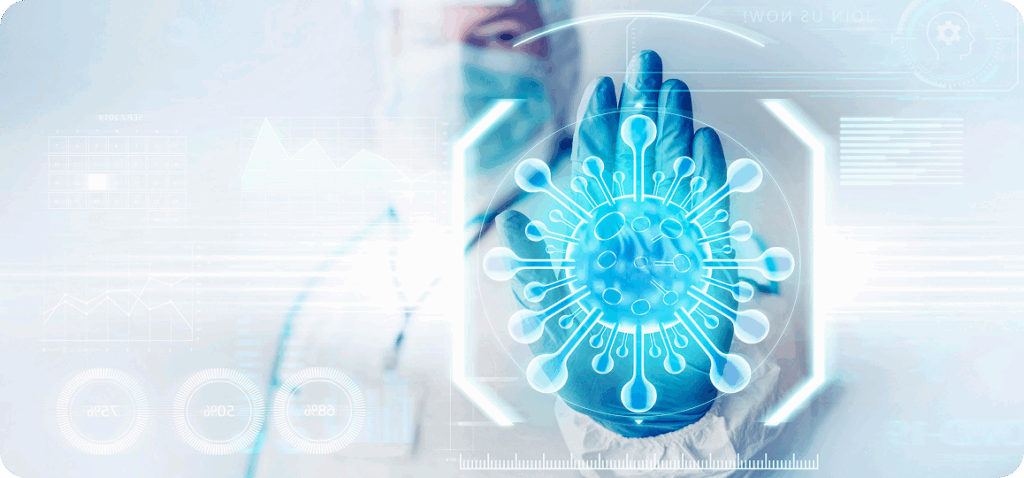
Personalized Medicine and Treatment
Big data allows for a personalized treatment on a broad scale. Traditionally, treatments were often one-size-fits-all, but today clinicians can analyze large datasets of genomic information, clinical outcomes, and even lifestyle data to tailor medical care to the individual.
By comparing a patient’s profile against vast databases, doctors can identify which therapies work best for that specific patient, thereby improving efficacy and reducing trial-and-error.
Leading institutions have launched big-data-driven personalized medicine programs. For instance, the U.S. Cancer Moonshot initiative leverages big data to analyze treatment and recovery records from millions of cancer patients, pinpointing which therapies yield the best results for each cancer subtype.
Insights from this program help oncologists match patients with the most effective treatments based on tumor genetics and similar cases.
In other words, big data tools cut through thousands of data points (genes, prior outcomes, trial criteria) to find optimal treatment options for a single patient in seconds. The business value is clear: more effective treatments sooner can mean better outcomes, fewer adverse events, and lower costs by avoiding ineffective therapies.
Patients receive care that is truly personalized to their condition, whether it’s a cancer patient getting a targeted therapy matched to their tumor mutations, or a cardiac patient receiving a therapy adjusted to their specific risk factors.
Over time, as healthcare accumulates even more genomic and outcomes data, personalized medicine will continue to grow more precise, potentially improving survival rates and quality of life across many conditions.
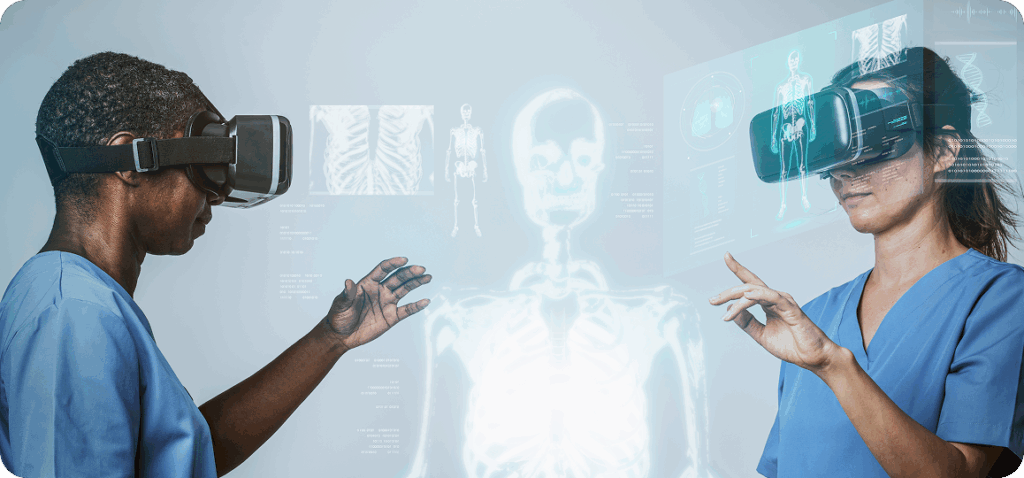
Real-Time Patient Monitoring with IoT and Wearables
The rise of the Internet of Things (IoT) in healthcare, from smart wearables like fitness trackers and smartwatches to at-home medical devices, has opened up a new dimension for big data.
Real-time patient monitoring generates continuous streams of health data that, when analyzed, can alert providers to issues in between regular visits. This is particularly valuable for chronic disease management and post-operative care.
By detecting warning signs early (such as an arrhythmia on a wearable ECG or a weight increase in a heart failure patient’s connected scale), clinicians can intervene before a minor issue becomes a full-blown emergency. Big data platforms aggregate and analyze these flows of remote patient data, often using AI to triage which readings are concerning.
Healthcare institutions have started to see tangible results from remote monitoring programs. The Cleveland Clinic, for example, has invested in remote patient monitoring for chronic respiratory and cardiac patients.
They equip patients with devices like connected spirometers and blood oxygen monitors that feed data into the clinic’s system daily. Clinicians note that daily monitoring can reveal subtle changes in a chronic patient’s condition, enabling early intervention before the problem requires an ER visit or hospitalization. This not only protects the patient’s health but also avoids the high costs of acute care.
Another case comes from UMass Memorial Health, which implemented an AI-driven remote monitoring program for heart failure patients. According to an American Journal of Managed Care report, this program reduced 30-day readmissions for heart failure by 50%.
As IoT devices become more prevalent, healthcare providers are poised to gather an unprecedented volume of real-world patient data. Analyzing this data in real time is now a core application of big data analytics, turning a flood of numbers into lifesaving action.
Conclusion
It’s evident that big data is driving a paradigm shift across healthcare. The business value is equally compelling, ranging from substantial cost savings and efficiency gains to improved patient outcomes and competitive advantage in a value-driven healthcare market.
For healthcare executives and managers, the challenge lies in moving from concept to practice. Implementing these big data applications requires not only vision but also robust data infrastructure, integration capabilities, and data governance to ensure privacy and accuracy. This is where choosing the right strategic partner becomes crucial.
Organizations like xLoop can serve as valuable allies in this digital transformation. As a digital engineering and big data consulting firm, xLoop specializes in building the kind of secure, scalable data platforms that healthcare providers need.
From establishing end-to-end data pipelines to deploying AI models, xLoop helps healthcare teams turn raw data into actionable intelligence, all while adhering to strict regulatory requirements and ethical standards.
With expertise in cloud data architecture, machine learning, and IoT integration, xLoop enables hospitals to implement predictive analytics solutions, real-time monitoring systems, and personalized medicine platforms that are both innovative and reliable.
Most importantly, xLoop emphasizes responsible data use: ensuring interoperability (so that healthcare data integration is seamless), maintaining patient privacy, and embedding AI in a way that augments clinical decision-making rather than replaces it.
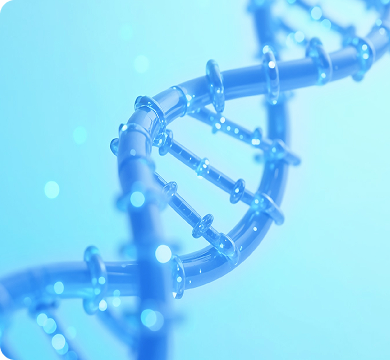
Schedule your free AI consultation with xLoop’s experts today and discover how we can help you leverage generative AI.
Table of Contents
Newsletter Signup
Tomorrow’s Tech & Leadership Insights in Your Inbox
About the Author
Shafay Islam
Shafay is a content and SEO strategist working at xLoop. He specializes in creating high-impact digital content, optimizing search performance, and driving brand visibility.
Discover More Insights
- All
- App Development
- Artificial Intelligence
- Cloud
- Cyber Security
- Data Management
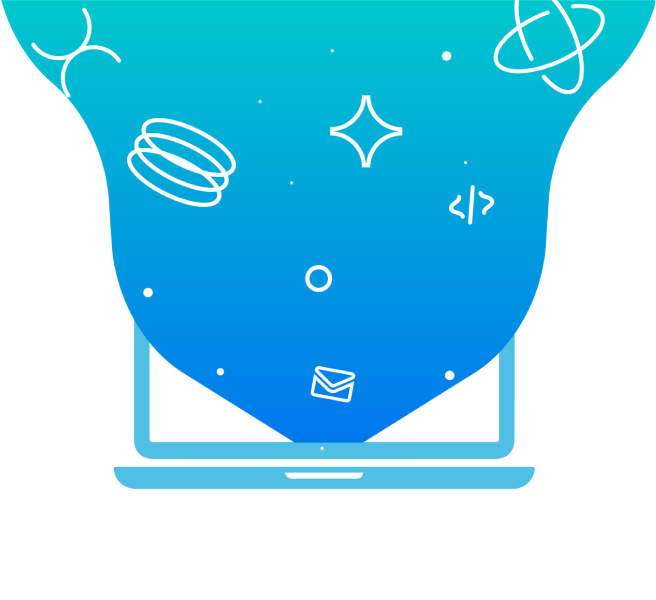